Introduction
Parkinson’s disease (PD) is a progressive chronic neurodegenerative syndrome typified by cardinal motor signs: tremor, rigidity, akinesia (brady kinesia) plus postural-instability. Since, no definitive test is existing for curing Parkinson`s, and the clinical-diagnosis is derived from, rooted as of the occurrence of clinical signs plus retort to anti Parkinsonian medicine.1 The majority of the conventional scale for evaluating disability and injury in P D is the Unified Parkinson’s Disease Rating Scale (UPDRS) stage III+ 2, and is rooted in founded on biased medical estimation of syndromes. Hence, necessitates quantifying P D characteristics scientifically in line, in sequence to advance”diagnosis”, define ”disease sub types”, observe this malady evolution or succession and also exhibit behavioral management effectiveness. 3, 4
D B S is a neurosurgical process for Parkinson remedy employs high frequency square-pulses to excite S T N neurons plus connected substructures and sub-regions of the human brain. Even though the means and methods of the deep brain simulator act are not comprehensive, exact neurochip implantation and stimulus coding and encoding might progress motor-signs also let for a decrease in anti Parkin sonian dosage medicine.5 But, the stimulus parameters are positioned by conventional guess-of-signs(GoS), also no phy-sio logical capacitative magnitude gauges are applied to maximize the value of stimulator for reducing motor-syndromes.6
Non invasive E M G plus kinematic gauges facilitate the scientific computation of neuro muscular functioning, action and movement progress therefore can be applied for computing the outcomes of deep stimulator of the brain, anti Parkinsonian medicine else supplementary curing.
Earlier experiments have shown that myography distinctiveness of subjects by this malady might transform because of owing to stimulator and anti Parkinsonian medicine in as a minimum 3 modes. Initially, the principal shaking frequency in the myo gram range, i.e., frequency-rage is augmented by stimulator of the brain followed by medicine.7, 8 The then, coherence amid myo graphy, hastening-acceleration (signifying tremor) is condensed – concentrated by stimulator, medicine in a latent state (devoid of cerebral or bodily pressures, strains, tensions, etc) also by rearward plus.8, 9
Lastly, period, stimulus-amplitude pulse-width of the primary distress disintegrate is amplified then the numerous distress fragments is compacted by the brain stimulator plus medicine through quick point-to-point movement-actions of jostle/prod10 followed withankle.11 On the other hand, yet the groupings of stimulator plus medicine cont standardize myo gram fissure breakage distinctiveness.11
Also previous experimental investigations have demonstrated that non linear and morpho logical techniques of myo graphy plus acceleration inferences in convolution through PCA approach (via execution of K-L transform) are greatly heklpful for discerning subjects by means of in the midst of the malady as of normal controls.12, 13, 14, 15 This consequence as of the reality that the myo gramss of subjects by Parkinson`s vary as of the myo graphs of normal controls, presetting spiny, inveterate anatomical-structures followed by the acceleration gatherings measurements depicting reliability.
But, this is not weathered whether approximating techniques of non linear dynamics followed by the K-L implemented latent variate-based (PC) approach are competent for computing outcomes of stimulator scientifically.
In this paper (experimental investigative research study) we demonstrate machine learning based unsupervised PCA and cluster tracking method for computing the outcome of deep brain stimulator in Parkinson`s by means of applying myo gram followed by kinematic investigation.
10 parameters capturing Parkinson movement disorder distinctive signal feature-manifestations were originally extrapolated as of iso metric myo graphy plus quickening signal-recordings, and those features (signals) were parameters of non linear dynamics, coherence amid myo graphy, quickening, plus stimulus-amplitude pulse-widths of increase of velocity (the acceleration). By employing the P C approach, the original parameters were transformed in to a lesser utmost at best 4 principal components. However, we considered first 3 PCs only.
At last, the results of brain stimulator were computed by observing the P C components in a low dimensional feature space (FS). The myo graphy plus increase of velocity data as of 12 Parkinson`s in the midst of STIMULI-ON and STIMULI-OFF, plus 12 normal healthy controls were employed for deducing the inferences. The outcomes of STIMULI on jostle (elbow) flexion also on (extension) porch actional-movements were inferred independently. The following table shows the clinical characteristics of the P D subjects.
Table 1
Clinical data of P D subjects
Hypothesis
Deep brain stimulation implants the micro neuro sensors or intelligent chips (i.e., microelectrodes) into an important area of the brain “substantia nigra (SN)”. The STN neurons are surrounded by SN. So, whether the implanted pulse generators damage the STN neurons or saves and reduce the motor symptoms.
Hence, the hypothesis of this study is that the built-up PC method is capable of computing the outcomes of D B stimulator on subjects with PD objectively. It follows that, the measurement characteristics of Parkinson`s are highly akin to measurement characteristics of normal controls (healthy) with “BRAIN STIMULATOR - O N” than with “BRAIN STIMULATOR - OFF”.
Materials and Methods
The following machine learning unsupervised computational techniques are applied in this study.
Latent variate factorial P Cs
10 micro signal features of sub thalamic nucleus neurons showed (subjects in Table 1), in Figure 1. We have implemented the Kerhnen Levin K-L transform in Mat Lab mainly for transforming the original correlated changeable-variables into un connected/correlated changeable-variables and for dimensionality reduction to decrease the number of changeables whilst observance as much as possible mostly informative concerning original-variables.
Figure 1
myo graphy plus IoV feature-values for a single normal person depicted with *asterisk and a diseased condition with P D brain stimulator-on /off depicted with circle(°).
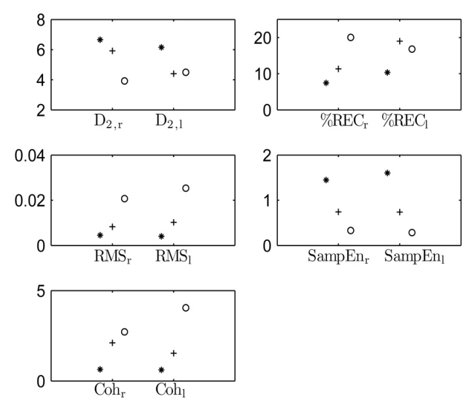
Upon computing, firstly 10 signal-parameters were positioned in sequence plus normalized to 0-mean plus-unit standard-deviation (SD) of normal`s to shape FVs for every-subject. Individual FV was shaped for every normal with 2-FVs for every-subject: 1 by “stimulator-on”, 1 by “stimulator-off”. Then, the dimensional-measurement of the FVs was decreased through P C-approach. Because in such given such move toward advance, the FVs were singularly decomposed (singular value decomposition) in to biased sum of orthogonal basis-vectors where ever the scalar weights were referred to as the dominant P Cs and they were the new and also un correlated un associated features and unconnected vectors.
We have chosen the basis-vectors were here by employing the FVs of normal’s, i.e., healthy-people. By choosing this selection, we ranged the features of normal`s within a single standard-deviation. So we clustered the normal`s into one conglomerate for comparative future study purposes and for computing the basis-vectors, we produced a feature-matrix which limited the FVs of normal`s within itscolumn. Later, we computed the correspondence-matrix which is the associated or connected correlatedof the feature-matrix and we also computed the corresponding dominant Eigen Vectors (EVs) as of that. 5EVs analogous to 5 highest magnitudes Eigen values were selected as “basis-vectors”. These 5 EVs given the 96% of the overall disparity in “FVs” of every normal. Therefore, every FV possibly will be fairly precisely formed as a biased sum of these 5 EVs.
Lastly, we computed P C s in a least square sense method for every normal and for every P D subject by “stimulator-on” and “stimulator-off”. The best P C s to discriminate among stimulator-on, stimulator-off-states plus connecting normal`s, subjects of Parkinson`s were preferred to deduce the inferences later on. It is this P C s that are offered in a 2D “feature-space” plus contrasted connecting “stimulator-on” plus “stimulator-off-states” as well as among Parkinson`s and normal`s. Consistent with the hypothesis of this study, in the FS the subjects shall be nearer to conglomerate of normal`s in the midst of “stimulator-on” than through the “stimulator-off”.
Principal Components
P C s was computed for every P Ds through the evaluated EVs. It is pointed that the P C 1 plus P C 3, i.e., first principal component P C 1 and third principal component P C 3 function and yields best in discerning among the brain-stimulator-on, brain-stimulator- off-states, plus among the P D subjects, normal`s.
The primary E V is the best mean-square-fit (MSF) for the FVs of normal`s. Thus, P C 1 (i.e. the coefficient of primary EV) expresses the stimulus-amplitude pulse-width of electro myo graphy plus increase of velocity features in connection with the mean-of-normal`s (MoNs). Observing the morphology-morphological-data (through-visually) of 3 EV, we may possibly distinguish, that P C 3 highlights the dissimilarity among the right-side(RS) and left-side(LS) changeable-variables.
Actually, the uni lateral on set plus importunate determined irregularity (,i.e.,asymmetry) of signs and syndromes hold the prognostics of Parkinson`s in connection to supplementary alike diseases and disorders or syndromes. 1 P C s 3, i.e., third P Cs in respect of the P C 1, i.e., all first3P C s of 12 normal`s plus 12 P D s through the stimulator-on, stimulator-off are showed in Fig. 2.
The P C values of 1 2 P D s are nearer to the middle-of-normal`s(MoNs), i.e., point0,0 in the FS through the brain-stimulator-on than by brain-stimulator-off, i.e., the electro myo graphy plus increase of velocity feature-values of them find nearer to normal`s also the plane distinctions shrink whilst the “brain-stimulator” is “ON” (Table 2).
Table 2
Feature-values (m e a n ± S D) for normal`s plus Parkinson`s whilst brain stimulator-on/off/
The expanse or detachment among the “brain-stimulator-on” along with “brain-stimulator-off”-states in the FS are extremely-individual. The distances to the center-of-normal`s (CoNs) are also given.
Figure 2
Principal components 3The third PCs a propos first principal components normal`s showed with *asterisk, Parkinson`s through the brain-stimulator-on showed with + sign, and brain-stimulator-off showed with circle sign.
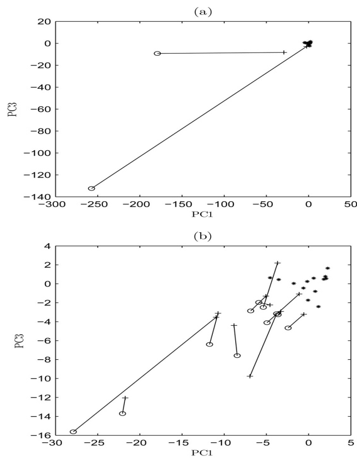
a. P D subjects 4,5plus normal`s, b. Left out P D subjects and normal`s. Brain-stimulator-on and off states of every Parkinson conditions are connected with a line.
Machine learning unsupervised PCA and Clustering algorithmic-techniques
Principal Component Analysis (PCA) is a statistical (mathematics) latent variate factorial (or factor) analysis (via dynamical systems) technique (based on heuristics i.e., trial and error based method) mainly to deduce the latent-dynamics (hidden features mainly for feature classifications purposes) from parallelly acquired higher dimensional STN neural-spiking-data. The PCA is a way of classifying patterns in data, and expressing the data in such a way as to underline their parities and disparities. As the patterns in data can be hard to find in data of higher-dimension, wherever the extra graphical-grid representation is not obtainable,
PCA is a powerful tool for analyzing massive data and high-end therefore high-speed hardware is necessary to process the volume of signals (data). PCA requires that the Eigen-values and the covariance matrix be formed. The Eigen-values obtained are unique for the entire set. Indeed, it turns out that the Eigen Vector (EV) with the highest Eigen-value is the principle component of the data set. The Eigen-vector with the largest Eigen-value is the one that will point down the middle of the data. It is the most significant relationship between the data dimensions. In general, once Eigen-vectors are found from the covariance matrix, the next step is to set them by Eigen-value, highest to lowest decreasing order in their magnitudes. The components with lesser significance can be ignored, as Eigen-values with small value do not result in much loss of data because they are negligible on electrical baseline (the zero line). If some of the components are left out, then the final data set will have fewer dimensions than the original one. If originally there are nEigen-vectors, then the final data set has onlydimensions.Taking Eigen-vectors, which are not ignored, and forming a matrix with Eigen-vectorsvectorA “FV”
FV = eig1 eig2 eig3… eign (1)
On forming the FV, get the transpose of the vector and then multiply it on the left of the original data set, transposed.
Last-data = row-FV × adjustment of row-data (2)
where row-FV is the matrix with the Eigen-vectors in the columns transposed so that the Eigen-vectors are now in the rows, with the most significant EV at the top, and the adjustment of the row-data adjustment of row-data is the mean-adjusted data transposed, i.e., the data items are in each column, with each row holding a separate dimension. The principal-component (PC) programme computes the mean-data-vector from all row-vectors win the initial data-matrix supplied to the PC-program. The residual data matrix was computed with the algorithm originally designed Jacobi’s method. 16, 17 Upon computing the residual data-matrix, and also the first three resulting principal-components are accumulated on to the computer hard disc, and the variance associated with each PC is verified and accumulated in conjunction with and the reason is to compute three principal-component-vectors (PCV’s), namely PCv1, PCv2, and PCv3 of a class of signals—waveforms.
The program arranges the data of a single signal into a matrix of the order m × n (m < n) by splitting the signal into m-segments each of length—n. Next, data minimization program determines PC coefficients a1, a2, and a3 and calls or invokes a function to operate on the following equation.12
X = G + a1.P1+ a2.P2+a3.P3+ error (3)
where, ‘X’ is the test phantom vector, ‘G’ is the mean of class of phantom vectors, P1, P2 and P3 are the first three PCs; a1, a2, and a3 are the principal components coefficients, such that the following error equation 4 is reduced
Results and Discussion
We presented a PC-based tracking method for quantifying the effects of DBS in PD by using surface EMG and acceleration measurements. The method was tested with EMG and acceleration data from 13 PD patients with DBS on and off, and 13 healthy age-matched controls. Detailed analyses are under discussed.
The electro myo graphy and the increase-of-velocity called accelerator (E M G and A C Cs) dimensional-measurements for the period of iso-metric-task(IMT) for a single normal person as well as one single P D diseased subject condition through the brain-stimulator-on and brain-stimulator-off are depicted in Figure 3.
Figure 3
Eelectro myo graphy plus increase-of-velocity(IoV-accelerator) signal-recordings of a single individual Parkinon diseased condition through the brain stimulator-off(left-side:LS) plus brain-stimulator-on in the center also one once single normal (right-side: RS) for the period of the iso metric – high force contraction of brachi-muscle.
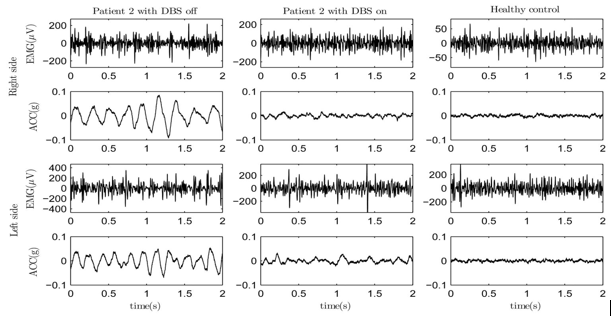
The outcomes of the iso metric at high force task levels (i.e., voluntarily muscle contraction at the high force levels i.e., isometric levels) depicted that the signal characteristics of 1 2 P D s were highly alike to the signal characteristics of normal`s through brain-stimulator-on than by brain stimulator-off. The distinctiveness examined in in myo gram, IoV signals amid P D subjects and normal`s, also among the brain-stimulator on/off-states recommended 3issues. At the outset, myo gram guages of Parkinson`s distorted and perturbed in to a highly multifaceted also restricted fewer chronic anatomical-structures owing to the brain stimulator. These chronicstructures are possible as a result of motoric-unit harmonization in hand-flexion and extensor muscles, which is trait for Parkinson`s.18 The outcomes of the brain stimulator i.e., deep brains stimulator have not been examined either by inferring the non linear myo graphy features. Also the stimulus-amplitude pulse widths promptness, timekeeping, reliability and the IoV gauges-measurement followed by the coherence amid electro myo graphy plus IoV decreased because of the stimulator and the outcomes submit to decrease in shaking palsy plus reliable through previous experiments. 7, 8, 9 Lastly, the elevation plane differentiations amid LS followed by RS changeable, i.e.,variables decreased through stimulator. The irregularity of signs and syndromes or feature manifestations is absolute basis for Parkinson`s. 1
The expanse among the stimulator-on, stimulator-off-states in FS, were extremely personage. Similarly, the progresses in prognostic diagnosis scores were exceedingly personage. Yet sturdy and robust variations in the overall U P D R S-stage-III+ cardinal motor-score did not result forever in sturdy variations in the inferred P C s visa-a-vis and vice-versa. It may be because of the information that the overall U P D R S stage-III+ motoric-score2 are a complex-score which containsof a huge number of sub scores. Hence, the sub scores are distinctive and divergent for dissimilar regions of the vertebra in singular actional-movement circumstances. In this paper, we inferred biceps bra chi (BB) muscles and arm actional-movement`s. In proportion to the outcomes, barely some of the P Ds achieve the normal`s in the FS through the stimulator-on. Actually, earlier authors have proved that the inferred electro myo graphy plus IoV features are possibly functional for discerning among Parkinson`s also normal healthy-people not considering of healing through medicinal drug. 13, 14 For one subject, towhom we gauged larger stimulusamplitudes and pulse-widths also high shaking-palsy/tremor through the stimulator on than via stimulator-off, was obviously auxiliary as of the normal`s in the midst of stimulator-on. While in clinical observations, this candidature shaking-palsy/tremor score was distincted larger by the stimulator-off than by the stimulator-on which proves that IoV gauge-measurements be able to give dissimilar in sequence concerning the shaking palsy/tremor than the experimental-eye-of clinical-diagnosing.
We have examined that the technique is perceptive to Parkinson`s through the linked and related shaking-palsy/tremor. The link among the shaking-palsy scores plus space to normal`s in FS is noteworthy p<0.0012highly significant statistically by a χ2@9.2859 with a 2 degree of freedom which is highly significant at5%.. Since shaking palsy/tremor appears within 80 % – 90% of Parkinson`s19 so is successfully decreased by the brain stimulator deep inside,5 and so the technique we used here is well suited for Parkinson`s.
Conclusion
As a conclusion, The voluntary contractions of the B B muscles at the isometric levels i.e., at the high force levels of electo myo graphy plus IoV gauges-measurements are highly employable in clinical sectors especially in neurocare centres for computing the outcomes of brain stimulators (deep into the brain)) on the neuro muscular function of Parkinson`s. These computations in convolution in the midst of the P C based tracking method might be employed to calculate the outcomes of the brain stimulator objectively/scientifically, cost effectively and non invasively, i.e., minimally invasive. In the future, the demonstrated approach might be t e s t e d, e x a m I n e d for assisting the fine-tuning of brain stimulator sets. Besides, the sensitivity of demonstrated-techniques to dissimilar forms of Parkinson`s ought to be predictable further cautiously in auxiliary experimental medical studies quantifiably.