Introduction
Parkinson’s disease (PD) is a chronic and complex neurodegenerative disorder characterized by the convolution of fundamental manifestations of its features such as cardinal manifestations: frequency-of-tremor, Bradykinesia, postural instability, and rigidity. It is getting a lot of interest due to the second most commonest neurological neurodegenerative disease after the cancer. It is one of the most common diseases among the old age population, whose age is more than 58 years. As the longevity of the people is increasing with the development of advanced technology, the size of the old age population above 58 years will be more and more in the coming years and the expected growth rate of PD will also follow the trend in the coming years. As the healthcare cost of PD is very high, it makes an impact on the economy of the developed countries. So, to avoid the economic impact as well as social impact, countries have started lot of research in recent years. The research has become more feasible with the invention of low deep bran stimulation of subthalamic nuclei in PD and low cost wearable sensors as well as powerful machine learning techniques. Wearable sensor-based method has lot of benefits such as low cost, light weight, energy efficient and also it functions without any external aids. So, the measurement process is much easier compared to the image-based process as well as other traditional methods.1 The gait analysis found to be most important tool for analyzing various neuro-logical disorders including PD. Spatiotemporal parameters has a huge contribution in the gait analysis as well predicting the PD. In this paper we have used multivariate analysis techniques with a machine learning approach. We have used various feature selection technique to select important features from those data. The selected features fed into different classifiers and the performance measures such as accuracy, precision and recall are evaluated and then by using this computed data the preliminary results are given.
The main pathology of Parkinson’s disease is present in the nigrostriatal system which is characterised by the degeneration of the dopaminergic neurons in the substantia nigra pars compacta. Substantia nigra pars compacta is a part of the basal ganglia which modulates the cortex and helps in fine tuning motor activities. There are two dopaminergic pathways involved form the striatum to the thalamus and the cortex- direct pathway which leads to stimulation of the cortex and the indirect pathway which inhibits the cortex. The dopaminergic supply from the substantia nigra pars compacta acts by D1 receptors which activate the direct pathway and the D2 receptors which inhibit the indirect pathway. Absence of these neurons leads to an increased firing from the subthalamic and globus pallidal interna neurons which leads to increased inhibition of the thalamic neurons and cortex and overall reduced movement.2 Figure 1 depicts the normal functioning of basal ganglia and the abnormality in idiopathic Parkinson’s disease.
The normal and abnormal function Basal Ganglia which is meant for our movement and motor control is depicted in Figure 2 (In case of the failure of the functioning of the globus pallidus neurons, the dysfunction of the Putamen component of the SNpc,pr, i.e., D2 and D1 receptors are failed)
The advent of treatment with initial levodopa followed by the armamentarium of various drugs but the medical treatment is fraught with appearance of various side effects such as dyskinesias and on-off phenomenon. The dopaminergic drive in normal patients is a continuous one and oral medications however cannot completely mimic the normal state with drug concentrations changing from trough to peak levels based on the time of consumption. Deep brain stimulation of bilateral subthalamic nuclei is an effective mode of rehabilitation in subjects (diseased conditions who are patients) with idiopathic Parkinson’s disease (PD). Accurate targeting and placement of micro-electro-neuro-chips (the microelectrodes) are paramount importance for optimal results after STN-DBS. Stereotactic assessment, intra-operative micro-electro-neuro-sensor recording and intra-operative stimulus effects have all been used in targeting, albeit the individual role of each modality is still not known.
Of the two, i.e., STN and GP, subthalamic-nucleus (STN) stimulation is associated with more drug reduction compared to globus pallidal stimulation. Subthalamic nuclei deep brain stimulation (STN DBS) involves placing two leads, one in each subthalamic nucleus with a pulse generator (i.e., the implanted pulse generators (IPG) placed under the skin on the chest.
The surgery is performed under stereotactic guidance –i.e., a stereotactic frame is placed on the head and the nucleus is identified on the magnetic resonance imaging (MRI) of the brain and the co-ordinates are obtained in the vertical and the horizontal planes, then with the help of these co-ordinates the leads are placed through a small hole on the scalp.
For optimal therapeutic efficacy of DBS, it is imperative to have accurate electrode lead placement.3 A small deviation in the electrode positioning may cause it to be misplaced in the surrounding structures such as the corticospinal tract, red nucleus, occumulotor nerve and other structures. Improper targeting may lead to various side effects such as speech disorders, visual deficits with diplopia, ocular deviations or motor stiffness. 3
MRI targeting
The problem with targeting subthalamic nucleus is that it is a small biconvex structure and not clearly identified on the MRI due to lack of contrast between the STN and the surrounding structures. 4, 5 The STN can be visualised on the MRI but other methods such as Lozano’s technique where a position 3 mm lateral to the superolateral border of the red nucleus is targeted have been studied and found to be effective areas for stimulation.6 As the MRI techniques are not absolutely perfect, use of electrophysiological techniques such as microelectrode recording from the subthalamic nucleus as well as intra-operative stimulation have helped in clearly demarcating the STN. Microelectrode recording can identify subthalamic neurons by their characteristic bursting pattern and their signals clearly identify the nucleus form the surrounding structures. On table stimulation is studied to ensure that the there is optimal benefit with the least side effects and this is the final test to ensure the correct targeting of the STN. All these techniques are normally used in combination during targeting, although the individual role of each modality is still unknown. Since the collected data contains many features, it is necessary to implement the feature selection and feature extraction methods to reduce the computational time as well as to compare the performance of the classifiers with the selected features. In this study, we used the MER with STN-DBS data (neural-signals) of Parkinson’s disease at a tertiary care hospital. The datasets were further preprocessed for modeling and feature selection and machine learning techniques are applied to measure the performances such as variance, root mean square (RMS), principal components (PCs) the effective diagnostic analysis. This study will help the clinicians to discover suitable patterns for early diagnosis of PD. The principal component (PC)-based tracking method is for quantifying the effects of DBS in PD by using electromyography (EMG) for muscle contractions. The parameters capturing PD characteristic signal features were initially extracted from EMG recordings. Using this approach, the original parameters were transformed into a smaller number of PCs. Finally, the effects of DBS were quantified by examining the PCs in a low-dimensional feature space. Clinical evaluation of subjects (the PD patients) showed that their motor symptoms were effectively reduced with DBS. The analysis results showed that the signal characteristics of 12 patients were 80% variant in our computation with DBS on than with DBS off. These observations indicate that the Machine learning PC-based tracking clustering method can be used to objectively quantify the effects of DBS on the neuromuscular function of PD patients. Further studies are suggested to estimate the clinical sensitivity of the method to different types of PD.
Biosignals are intelligent biological biomedical signals which are massively curved data streams helping highly to the development of frontier technologies’ especially in health care sectors medical systems for clear understanding of the disease and predicting symptoms of the disease in early stage. Such signals are modeled and designed by scientists and engineers (trans-disciplined). Parkinson`s disease (PD) is a second most chronic progressive commonest neurodegenerative disorder, characterized by cardinal features of tremor, Bradykinesia, rigidity and postural instability.1, 2, 7, 8 The disease is linked with different anomalies in brain function and structural-composition. The pathological hallmark of the disease is a bottomless failure of nigrostriatal-dopamine-cells and an accrual intracellular inclusion referred to as Lewy-bodys that are encompassed α-syncluin aggregates. In act with dopaminergic-cell-loss, several indeed many other neurotransmitter-systems as well degenerate and later on Lewy-body pathology too spreads to the motor-cortex. This makes PD prototypical systems disorder, which can be completely tacit merely when investigating experimentally molecular, anatomical-structural and functional anomalies at the level of brain-net-works. Therefore, the search for optimal cure is on for the past two centuries since the time it was first described by James Parkinson.9 However, scientists established that the malady arises from insufficient quantities of the neurotransmitter-dopamine in an important area of the brain that controls movement, the basal ganglia.
Perhaps PD best known for its tremor slows and stiffens movements.10 From the 1940s during the 1960s, surgeons combated the disorder by razing areas of the brain, chosen more by heuristics based trial and error method than by a lucid perceptive of neural misconduct. The so-called lesions created by these operations repeatedly distributed extravagant and steady-effects, thwarting the tremor and, to some degree, further features or symptoms of this malady. Yet minor misplacement, nonetheless, brought difficulty rather than advantages. Such break was enduring, as dead tissue could not be revived. The 1960s broke open a new therapeutic-treatment for the malady and primarily set aside the surgical-era.
One of the most effective treatments for PD is deep brain stimulation (DBS) of the subthalamic nucleus (STN). The procedure involves the surgical implantation of stimulating electrodes into the STN and provides a unique opportunity to record in vivo the related neuronal activity, through microelectrode recordings (MERs) of high spatio—temporal resolution. However, the optimal placement of the stimulation electrode continues to be a challenge, possibly due to the neuroanatomic variability within the STN sensorimotor area.3 MERs have been used before to enhance our understanding of how STN neurons function and classify possible mechanisms for DBS in PD. MER-based algorithms have also been developed to categorize and detect the sensorimotor area within the STN by using both the high and low-frequency content of the recorded signals.11 The high frequencies of the MER signal include both the electrical action potentials from neurons located closest to the electrode tip (typically at a distance of less than 100–300 μm) as well as smaller sub-noise level spikes from nearby neurons known as “background-unit-activity” designated as BUA. The combinations of these two signals are referred to as “multiunit-activity” referred to as MUA. The lower frequencies of the MER signal correspond to the local field potential called LFP potentials, which reflect the cumulative activity of a population of neurons within a better diameter from the electrode tip (around 0.5mm–3mm). So far, most of the MER based analyses of the STN have focused by a series of scientists3, 4, 5, 6, 12, 13, 14, 15, 16, 17, 18, 19, 20, 21, 11 on the gross automatic detection of the STN borders.
Amirnovin et al3 conducted the study on microelectrode-signal-recording (MER) in targeting subthalamic-nuclei (STN) in 40 Parkinson candidates (i.e., Parkinson diseased subjects). The predicted location (with the preoperative deep brain stimulation with the magnetic resonance imaging, DBS MRI) was used in 42% of the cases; however, in the remaining 58% of the cases it was modified through MER (MER with STN-DBS). By applying MER technique, an average pass through the subthalamic-nucleus (STN) of 5.6 mm was attained and evaluated to 4.6 mm if the central-tract was selected as per the MR-imaging. Application of microelectrode-recording augmented the path through the subthalamic-nuclei by 1 mm, increasing the likelihood of implanting the microelectrodes with the deep brain stimulator squarely in the STN, which is relatively an elfin or petite target. Bour et al6 studied the outcome of MER in 57 PD patients with STN-DBS and deduced the following inferences. For the subthalamic-nucleus, the central-trajectory was chosen for inserting-microelectrodes in 50% of the cases, the channel selected had the longest segment of STN with the MER activity in 64% of STN DBS cases. In case the central electrode was selected for embedding the innocuous micro electrode, this was also the channel with the best microrecording in 78% for STN. The final electrode-position or electrode-point in the STN, if not placed in the central-channel, was often more lateral than medial to the computed—evaluated target ten percent (10%, i.e., 10 patients / 98 patients) lateral; six percent (6%, i.e., 6/98) medial and frequently more anterior 24% (i.e., 22/98) than posterior 10% (10/98). The mean and standard deviation (SD) of the deepest contact-point with respect to the magnetic resonance imaging (MRI)-based target for the STN was 2.1 mm ± 1.5mm. The goals of our study was to unveil the significance of intraoperative microelectrode recordings of neural signals and elucidate their predictive role in terms of the response to STN-DBS, to identify the MER signal characteristic discharge patterns (or signatures) of STN that correlate with improved symptoms of PD as well as movement-related activity (MRA), to experimentally investigate the correlation of microrecording with the final-tract chosen during bilateral subthalamic-nuclei deep brain stimulation.
The best trajectory was considered as the one with the longest STN recordings and detectable MRA and finally to quantify the efficacy of MER with STN DBS using principal components based tracking method. The surgical procedure for placing the stimulating microelectrode in the track MERs has a 1-mm precision, both horizontally and vertically.5 Intraoperative current stimulation (60μs pulse width, 130 Hz frequency, 0.5- to 5.0 volts amplitude) verified the short-term clinical improvement and identified possible side effects.
Although anatomical structural organization provide some clues as to what might be the function of basal ganglia circuits in PD patients, albeit the inference of function from anatomical structure is exploratory. One investigative approach to studying-the-function of an area-of-the-CNS in particular substantia-nigra (SN) is to acquire the STN neurons with extracellular MER in locally anesthetized PD patients.13, 14, 15, 16 Other approaches involve inferences of neuronal signaling from imaging studies of blood flow and metabolism, or of changes in gene expression. By sampling the signal of a part of the brain during behavior, one can gain some insight into what role that part might play in behavior. Neurons within different basal ganglia nuclei have characteristic baseline discharge patterns that change with movement.17, 18 In this study, we followed the MER approach. Keeping this in mind, a retrospective study was carried out at tertiary care NIMS Hospital and research center (Hyderabad, Telangana State TS, South India) with a dedicated movement disorder unit in Neurology department of NIMS and biomedical eng. Twelve subjects with diagnosis of PD as per United Kingdom Parkinson disease society brain bank criteria are included in the study.
In regard to the PD symptoms prediction, Kyriaki Kostoglou, et al4 hypothesized that a data informed combination of features extracted from intraoperative MER which can predict the motor improvement of PD patients undergoing DBS surgery. Sang Jin Kim, et al,5 hypothesized that STN-DBS will improve long term potential (LTP) like plasticity in motor cortex of PDs. Zuan He12 hypothesized that DBS works by reducing the level of synchronization among the neuronal firing patterns within the target site and proposed a desynchronization-based closed strategy for the generation of DBS input (nonlinear delay feedback stimulation NDFS). Sabato S, et al.21 Investigated the therapeutic mechanisms of HF DBS in PD by developing a computational simulation model of cortico-BG thalamo-cortical loop in controls and Parkinson conditions under the effects of DBS at some frequencies. Further they found that the DBS infused in the loop educes subtle neural changes that travel along multiple pathways with different latencies meet in striatum. In a US based study.21, 11 high frequency (HF) DBS is clinically recognized surgical therapy to treat Parkinsonian movement disorders, but its mechanisms remain hazy. Recent hypotheses imply that the therapeutic value of HF-DBS stalks from enhancing the regularity of the firing patterns in basal ganglia.
Literature Survey
Parkinson’s disease (PD) is one of the most commonest neurodegenerative chronic movement disorders, is caused by damage to the central nervous system (CNS).22, 23, 24, 25, 26, 27, 28, 29, 30, 31, 32, 33 The manifestations or symptoms analogous to cardinal motoric features of PD have been mentioned as “Kampavata” in ancient Sanskrit Vedic Hindi documents. Parkinson`s disease was termed “Shaking Palsy” by the Galen, a famed Roman physician. From then on, the idiom or term `Shaking Palsy` has been used by physicians in modern medicine. The first modern scientific research on PD was done by the British doctor James Parkinson. 34, 35, 36, 37, 38, 39, 40, 41, 42, 22, 43, 23
James Parkinson FGS (11 April 1755 – 21 December 1824) was an English surgeon, apothecary, geologist, palaeontologist and was a political activist. He is best known for his 1817 work “An Essay on the Shaking Palsy,” in which he was the first to describe `paralysis agitans`, a condition that would later be renamed Parkinson's disease by Jean-Martin Charcot. Parkinson studied six PD patients and documented a few PD symptoms for the first time. About 50 years later, Charcot, a French physician, studied some neurologic diseases, especially multiple sclerosis (MS) and PD. Jean-Martin Charcot was the first person to distinguish MS and PD at a time when most neurological diseases were collectively called shaking palsy. Charcot named the disease “Parkinson’s Disease” after extensive studies done by James Parkinson on PD.17 Charcot’s researches between 1868 and 1881 are considered as a landmark in our knowledge of PD.44 The studies on PD continued and many other researchers made contributions to the understanding of PD. Tretiakoff42 found an association between cell loss in substantia-nigra and tremor,42 and this result was confirmed by Hassler.45, 46 For many decades, scientists and researchers hard slogs on alleviating PD symptoms were unproductive, but in the1940s and 1950s pallidotomy and thalamotomy were introduced as two effective surgical methods for the treatment of PD.47, 48 In the 1960s, scientists discovered that dopamine is decreased in PD patients’ brain.16 In the 1961–1962, discovering some drugs like Levodopa that increases the amount of dopamine was a turning point in PD history.49 Besides the progress in drug therapy, the neurosurgical therapy for PD attracted the attention of some researchers. In the 1990s, brain surgery studies led to the development of new horizons in PD treatment, and deep brain stimulation (DBS) was introduced as a treatment method for the advanced PD stages.40 Despite all of the studies on PD, the formation mechanism of its symptoms remained unknown. It is still not obvious why damage only to the substantia nigra pars compacta (SNc), a small part of the brain, causes a wide range of symptoms. Moreover, the causes of brain damages remain to be fully elucidated.6, 19
PD is among the most prevalent neurodegenerative diseases, ultimately causing disability and reduction in quality of life.50 The real cause behind PD is still unknown, and hence, there is no cure for the disease yet.19 As a complex disorder, PD often causes devastating symptoms. Its main motor disturbances include Bradykinesia, postural instability, rigidity, and resting tremor.17
The prevalence of PD is estimated at 160 per 100,000 in the general population, and annual incidence is 15–20 per 100,000.51 PD is a neurodegenerative disorder which is related to age as well. Diagnosis rate of subjects below the age of 40 is <10 %, with its prevalence among the population over the age of 65 is about 1 % and rises to 3 % among the population over 85 years of age.52 In most of the per-formed studies, it appears that PD is relatively more common in men than in women, ranging from a 1.2:1 ratio up to a ratio of 1.5:1.10 Despite PD affecting all ethnicities, observations demonstrate differences both in incidence and prevalence among them. For instance, North American and European people of Caucasian ancestry show a high prevalence rate, while the rate among Chinese and Japanese populations is moderate, and prevalence is lowest among African-Americans. Because of the complications associated with PD, it can increase the mortality rate. 53
The cost of the illness increases with the PD progress. This creates an economic burden which affects the patients, society, and healthcare system alike. Estimating the overall cost depends on the country. The annual total cost in the UK has been estimated at between £449 million and £3.3 billion.18 This figure for the annual economic cost of the disease in the USA, both directly and indirectly, has been estimated to be nearly $25 billion based on the data pro-vided by the PD foundation. 14
The controlling ways of the disease progression and neuroprotective treatments are not fully discovered. All of the symptoms and their growing process are also not certain
Yet.54 Therefore, the study of PD remains crucial for the scientists, but studies regarding different fields of PD are in progress. Some researchers focus on the unknown symp-toms of PD. These symptoms are divided into two main groups: motor symptoms and mental symptoms. Movement disorder symptoms are known to some extent, whereas the mental symptoms are vague.55 Other researchers have tried to find out the causes of the disease. Moreover, it is not clear when the destruction of the basal ganglia (BG) is started. It is also unknown whether it is predictable or not. Generally, PD is dependent on vague factors such as genetic disorders, free radicals, and environmental factors.5, 11 Some researchers seek to offer new and more appropriate therapies and drugs. Although Levodopa therapy plays a crucial role in the early stages of the disease and sup-presses its symptoms, high doses of the drug seem to be needed with the development of the disease.17 Gradually, the effects of L-Dopa will decrease in some stages of the disease. Afterward, additional medications or non-pharmacological treatments such as DBS are required.54 Major research groups are trying to diagnose the disease in its early stages, for it can control the progress of PD, and as a result the severe stages of the disease can be postponed. This process can also be very effective for improving the patients’ living conditions.56 Some other study groups look for a better understanding of PD and the mechanisms of its symptoms.12 The causes of a wide range of symptoms collectively identified as PD are still unknown.19 Understanding the generation mechanism of the symptoms is very useful for their improvement.
Some researches follow the PD computational studies that can open the extant horizons for the disease understanding. Computational studies have two distinct fields. One group includes the computational analysis of the symptoms, while the other tries to present proper models for the symptoms. Although there are similarities between these two, their objectives are quite diverse. In the computational analysis studies, one of the disease symptoms is recorded in a computational manner, and some features of the symptoms are extracted through mathematical analysis and signal processing methods, leading to a better understandings of the symptoms’ behavior. Computational analysis of the symptoms can be very valuable in early diagnosis of the disease. It can also be used to quantify different stages of PD. Obviously, various treatments are required at different stages. Other computational studies are related to the behavior modeling of the disease symptoms. Computational modeling may play an important role in understanding the behavior and performance of PD. The brain structure is very complex, and its function still remains unclear. In the diseased state, the complexity of the structure advances due to the change in the neurotransmitter behavior in the brain.
Figure 3
Block diagram of computational models, a. model black box, b model gray box I/p – Input, O/p data – Output data
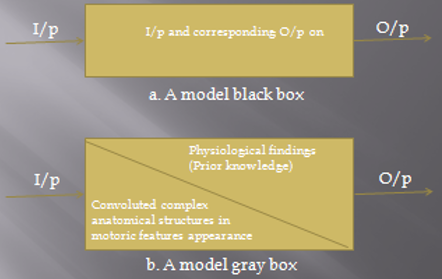
Computational modeling may help us figure out brain functions. Modeling studies include two main groups: black-box models and gray-box models. In black-box modeling approach, regardless of the system information, the symptom is only considered as the model output. The aim of the black-box modeling is finding a mathematical relation, which is able to produce behaviors, similar to the disease symptoms. Such a model can be used in distinguishing the behavior of the symptoms and long-term predictions. The gray-box modeling approach considers the involved structures in the symptoms appearance as well as the final disease symptoms. In gray-box modeling, the purpose is to produce a relation for symptoms, while the characteristics and parameters of this relation should be similar to physiological findings related to the symptoms producing structures. So, in this type of modeling, each relation that is able to simulate the disease’s symptoms is not considered; how-ever, just the relations, which have good similarities with the real system behavior, are considered. Such a model can be useful in our understanding of the involved structures in the disease diagnosis and their role. In gray-box models, the disease process and even medical or non-medical treatments can be investigated in order to identify their effectiveness. A block diagram of black- and gray-box models is presented in Figure 1.
As discussed above, there are different fields of study in PD. Some computational studies have been done in past three decades concerning the dynamic behavior of the disease. In this review paper, we will attempt to investigate conducted computational studies on PD. For this purpose, in Sect. 2 we introduce some of the computational analysis studies on PD. Such studies are necessary for presentation of a mathematical model. Section 3 reviews the black-box models. In Sect. 4, we present gray-box models of PD that consider some physiological findings. In Sect. 5, we provide an investigation of reviewed models. Finally, in Sect. 6, we draw some conclusions about difficulties in modeling and delineate future works.
Parkinson`s disease symptoms and computational analysis
Disease symptoms signal recordings are necessary in computational studies. After appropriate signal recording, it should be processed in order to investigate the disease output behavior. These signals and their analysis are required for the modeling. Although some studies perform computational analysis on the signals resulting from disease symptoms, they do not model the disease itself. Since PD computational models will be reviewed in the following section, it is better to generally review recording methods for disease symptoms.
In most cases, computational analysis of the disease is implemented in regard to the movement symptoms. The main symptoms of the disease, which are highly focused on, include: tremor, finger tapping, gait disturbances, electroencephalography (EEG) signals abnormalities, and balance disorder. More focus has been on tremor and gait disturbances due to their simple needed recording method.
Studies in computational analysis can help us separate the patients from healthy people. This is very crucial, because there is no routine test to clinically identify PD patients. Such tests can be very helpful in early diagnosis of the disease. In general, when the disease is diagnosed by a physician, about 50–80% of SNc neurons are already dam-aged.17 Diagnosis in the first stages can prevent further damages. Using computational analysis of symptoms, we can define a class for healthy and patients subjects that can be a base for disease diagnosis or even its early diagnosis. In addition, different classes could be defined for different stages of the disease. Obviously, different stages require various treatments.
Some studies focus on the EEG signals of various parts of the brain that obtained from the centers involved in the disease. In this manner, the differences between the behavior of the control group and the PD group are examined. The SNc is a small part of the BG that actually consists of a very small part of the brain. This makes the analysis of EEG and spike trains very vague. Therefore, it requires an exact recording method. The same is true for the magnetic resonance imaging (MRI), functional magnetic resonance imaging (FMRI), positron emission tomography (PET), and single-photon emission computed tomography (SPECT) images, which clearly show the differences in brain behavior between normal and Parkinson’s groups. It also requires complicated processing and determination of an accurate target.57
Hausdorff et al. have investigated the gait problems. His group studied the gait behavior and introduced a stable long-range correlation in the stride interval of healthy people.58, 59 They concluded that the fractal structure is affected by aging and neurodegenerative disorders. 60
Related Work
Due to the significancy of the work and its importance to the areas and fields of computer science, the artificial intelligence (AI) machine learning techniques, are widely employed because of its effectiveness in various fields. Some previous works related to these fields are as follows. The performance improvement and the avoidance of over-fitting is one of the most important criteria for decision making can be achieved by feature selection techniques.7 The role of the feature selection techniques is to remove the weakly associated features and keep the strongly associated features.8 Statistical signal processing based algorithm feature selection technique was used to diagnosis the PD using the voice datasets by removing the weakly associated features from the dataset that ultimately provides good accuracy.9, 10 Machine learning techniques have been successfully used in different fields as well as in the field of PD. Most of the machine learning techniques were used as a prediction model for detection as well as diagnosis of PD. Different stages as well as severity of the PD was studied using ML techniques. From computation, it was found that the clustering gives clinically accepted 80% variance and 96.45% confidence levels.10 Drawing movement of PD patients as well as normal controls was well distinguished using machine learning techniques. An average accuracy of 91% was found while distinguishing these two groups.3 PCA- based tracking method is successfully used to choose first three PCs which effectively reconstruct the original data and its corresponding features extractions.4, 5
Objectives
To study the effectiveness of lead position with micro-electro-neuro-sensor recordings in concluding and determining Subthalamic Nuclei for embedding the Deep Brain Stimulation (STN DBS) microchips (electrodes) during bilateral STN-DBS.
To acquire the implantation of microelectrode recording (MER) signals data of subthalamic nucleus (STN) neurons via deep brain stimulation (DBS) in Parkinson`s disease (PD)
To find out the variance of 12 PD subjects micro-electro-neuro-sensors of STN signals with DBS
To study the correlation of microelectrode recording with the final tract chosen during bilateral STN DBS performed at a specialized tertiary care centre in South India.
To compute the MER signals of subthalamic nucleus neurons with deep brain stimulation in Parkinson`s using multivariate techniques - principal component analysis (PCA, a statistical mathematical latent variate factorial or factor analysis) and clustering method with machine learning approach
The flow of the study is organized as follows: Section 1 is the introductory part which gives the background of the study, literature survey/ related work and computational analysis of Parkinson`s disease. Section 2 gives goals of the study which are objectives. Section 3 describes about the methodology - methods. Section 4 describes about the preliminary results and discussions. In Section 5 inferences were drawn and conclusions deduced.
Methodology
Deep brain stimulation (DBS) is an established therapy foradvanced Parkinson’s disease (PD), with relatively strictinclusion criteria to obtain optimal improvement of motorfunction.13, 51 However, the effects of DBS on non-motor manifestations, such as the new onset of cognitive de-terioration,61 have become a critical issue in recent years.
The data flow and system flowchart model of the PD model architecture is shown in Figure 1.
A retrospective study was carried out at a tertiary care multi-specialty hospital with a dedicated movement disorder unit and computer science and biomedical engineering team from South India. Fifty two patients with diagnosis of PD as per United Kingdom Parkinson disease society brain bank criteria were included. All the patients were willing to undergo the procedure and fulfilled the following criteria to be eligible for STN-DBS i.e., they had disease duration of 6 years or more, good response to levodopa, able to walk independently in drug “on” state and had normal cognition. All PD patients who were wheelchair or bed bound, had dementia or severe psychiatric disturbances were excluded from this study. Computer Aided Stereotactic Functional Neuro Surgery was performed in all by a qualified neurosurgeon. Stereotactic targets were acquired using a specialized system with a stereotactic frame (CRW) which has a luminant MR localiser. The targeting was performed according to Lozano’s technique – 2mm sections are taken parallel to the plane of anterior comissure-posterior commissure line and at the level with maximum volume of red nucleus, STN is targeted at 3 mm lateral to the anterolateral border of red nucleus.
The co-ordinates are entered into a stereo-calc software which gives the co-ordinates of the STN. Another neuro navigation software –Framelink is also used to plot the course of the electrodes and to avoid vessels. The surgery is performed with two burr holes on the two sides based on the co-ordinates. Five channels with are introduced with the central channel representing the MRI target while medial (nearer the centre) and lateral (away from the centre) are placed in the x axis while anterior(front) and posterior (back) are placed in the y axis to cover an area of 5 mm diameter.
Intra-operative recording was performed in all 5 channels. All five microelectrodes are slowly passed through the STN and recording is performed from 10mm above to 10mm below the STN calculated on the MRI. STN IS identified by a high noise with a large baseline and an irregular discharge with multiple frequencies. Figure 5 shows the microelectrode recording which is obtained from the STN
Figure 5
Picture of the microelectrode recording –the panel in the top left shows recording in a single level in a single channel; the panel in the bottom left shows the recording in the central channel over 11 mm and shows the typical firing pattern with irregular firing and broad baseline noted from -1.00 level; the top right shows the typical histogram frequency and the fastfourier transformation graphs of a typical STN neuron; the bottom right shows the same in a linear fashion
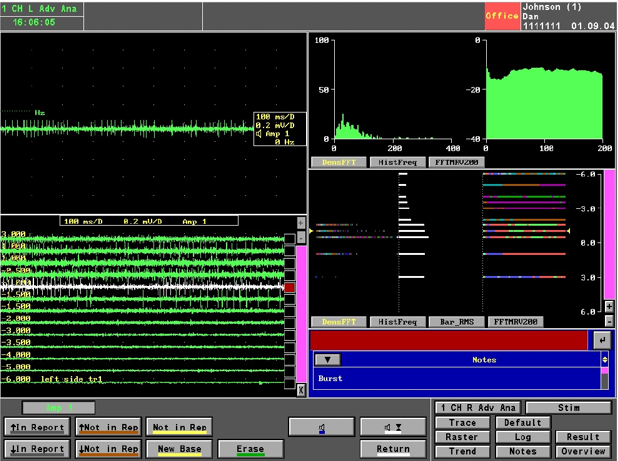
The channel with maximum recording and the earliest recording were recorded on both sides Intraoperative test stimulation was performed in all channels from the level at the onset of MER recording. Stimulation was done at 1mv, 3mv to assess the improvement in bradykinesia, rigidity and tremor. Appearance of dyskinesias was considered to be associated with accurate targeting. Side effects were assessed at 5mv and 7mv to ensure that the final channel chosen had maximum improvement with least side effects.
Correlation was assessed between the aspects of MER and the final channel chosen in 46 patients (92 sides).
Variance
For a given population of size N (12), the
Where
s2 =
Covariance matrix
Given
where,
giving the usual variance
where,
The mean µ [σ2] and the variance Var [σ2] of σ2 are calculated as
Var [σ2] = µ [(σ2 − σ¯2)2] = Var[ε]................(7)
Since x is expressed as the multiplication of σ and white Gaussian-noise W passed through H, the conditional distribution of x given σ2 is Gaussian distribution with a mean of zero and a variance of σ2:
Eigen-Values
Each Eigen-value is paired with a corresponding so-called Eigen-vector (or, in general, a corresponding right Eigen-vector and a corresponding left Eigen-vector; there is no analogous distinction between left and right for Eigen values). The decomposition of a square matrix A into Eigen-values and Eigen-vectors is known in this work as Eigen decomposition, and the fact that this decomposition is always possible as long as the matrix consisting of the Eigen-vectors of A is square is known as the Eigen decomposition theorem.
Let A be a linear transformation represented by a matrix A. If there is a vector
for some scalar
Let A be a
with Eigen-value
which is equivalent to the homogenous system.
Equation (12) can be written as
where, I is identity matrix. A linear system of equations has nontrivial solutions if and only if the determinant vanishes, so the solutions of equation (13) are given by,
This equation is known as the characteristic equation of
Jacobi’s Method
To compute Eigen values and corresponding Eigen vectors, in our computation, we adopted the Jacobi method is a method of solving a tridiagonal matrix equation with largest absolute values in each row and column dominated by the diagonal element. Each diagonal element is solved for, and an approximate value plugged in. The process is then iterated until it converges. It may be noted here that the mathematical procedure is truncated due to restriction on the length of the paper. The algorithm reviewed from numerical algorithms group (NAG) routines Bath Information and Data Services (BIDS), University of Bath, UK, and from the numerical recipes in C/C++ text (Cambridge University press 19).
It is well known that sinusoidal are convenient basis for analytical purpose but they are not efficient for processing complex waveforms, such as STN, EEG and EMG signals. For such signal processing, principal component analysis (PCA) which are in some way resembles basis functions basis vectors having orthogonality (orthogonal-functions) is employed.
Principal Component Analysis
Principal Component Analysis (PCA) is a statistical (mathematics) latent variate factorial (or factor) analysis (via dynamical systems) technique (based on heuristics i.e., trial and error based method) mainly to deduce the latent-dynamics (hidden features mainly for feature classifications purposes) from parallelly acquired higher dimensional STN neural-spiking-data. The PCA is a way of classifying patterns in data, and expressing the data in such a way as to underline their parities and disparities. As the patterns in data can be hard to find in data of higher-dimension, wherever the extra graphical-grid representation is not obtainable, PCA is a powerful tool for analyzing massive data and high-end therefore high-speed hardware is necessary to process the volume of signals (data).
The algorithmic analysis is as follows. PCA requires that the Eigen-values and the covariance matrix be formed. The Eigen-values obtained are unique for the entire set. Indeed, it turns out that the eigenvector with the highest Eigen-value is the principle component of the data set. The Eigen-vector with the largest Eigen-value is the one that will point down the middle of the data. It is the most significant relationship between the data dimensions.
In general, once Eigen-vectors are found from the covariance matrix, the next step is to set them by Eigen-value, highest to lowest decreasing order in their magnitudes. The components with lesser significance can be ignored, as Eigen-values with small value do not result in much loss of data because they are negligible on electrical baseline (the zero line). If some of the components are left out, then the final data set will have fewer dimensions than the original one. If originally there are nEigen-vectors, then the final data set has onlydimensions.
Taking Eigen-vectors, which are not ignored, and forming a matrix with Eigen-vectors in the columns forms a feature vector. A feature vector is a vector consisting of multiple elements or features which gives the characteristics of the object.
Feature-vector = eig1 eig2 eig3… eign (15)
On forming the feature-vector, get the transpose of the vector and then multiply it on the left of the original data set, transposed.
Final-data = row-feature-vector × adjustment of row-data (16)
where row-feature-vector is the matrix with the Eigen-vectors in the columns transposed so that the Eigen-vectors are now in the rows, with the most significant eigenvector at the top, and the adjustment of the row-data adjustment of row-data is the mean-adjusted data transposed, i.e., the data items are in each column, with each row holding a separate dimension. The principal-component (PC) programme computes the mean-data-vector from all row-vectors win the initial data-matrix supplied to the PC-program. The residual data matrix was computed with the algorithm originally designed Jacobi’s method.19, 20 Upon computing the residual data-matrix, and also the first three resulting principal-components are accumulated on to the computer hard disc, and the variance associated with each PC is verified and accumulated in conjunction with. The reason is to compute three principal-component-vectors (PCV’s), namely PCv1, PCv2, and PCv3 of a class of signals—waveforms. The program arranges the data of a single signal into a matrix of the order m × n (m < n) by splitting the signal into m-segments each of length—n. Next, data minimization program determines PC coefficients a1, a2, and a3 and calls or invokes a function to operate on the following equation.14
X = G + a1.P1+ a2.P2+a3.P3+ error (17)
where, ‘X’ is the test phantom vector, ‘G’ is the mean of class of phantom vectors, P1, P2 and P3 are the first three PCs; a1, a2, and a3 are the principal components coefficients, such that the following error
is reduced.
Those coefficients which distinguishes a test-turning-point-spectrum by a point in 2 or 3 dimensional-vector-spaces. As a result, the PC coefficients let a representation of STN sequence, which can be plotted in a two and/or three dimensional-space. Figure 6 obtained with MER. The STN was detected by a high-noise with a larger electrical baseline (the zero-line) and irregular-discharge-patterns of multiple-frequencies.
Figure 6
The MER signal patterns of STN neurons for various-depths: -4mm to +10mm. It can be observed that the thin part of the STN is recognized by amplifying background-noise and a rise in discharge pace differentiated by the rhythmic-bursts-of-activity with higher-frequencies (neurons 3, 4, 5 and 6). The negative (-4 to -1)) values correspond with positions above the MRI-based target. Deeper layers of the STN show a more irregular-high-frequency discharge-patterns.
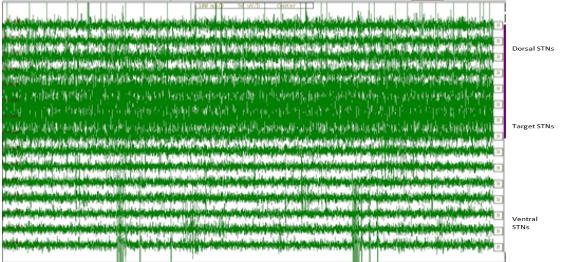
The STN was clearly characterized from dorsally located zona-incerta and lenticular-fasciculus (field H2) by an increase (abruptly) in background-noise level and increase in discharge rate typically distinguished by rhythmic-bursts-of-activity with a burst-frequency between 20Hz to 35 Hz. Intra-operative recording was performed in 5 channels. Five microelectrodes were passed slowly throughout the STN and recording was performed from 10 mm dimensions above to 10mm dimensions below the STN planned the on MRI. The STN was identified by a high-noise with a huge electrical baseline and irregular-discharge-patterns with multiple-frequencies. Figure 7 shows the microelectrode recording and the Fast Fourier Transform (FFT) which was obtained from the STN.
Figure 7
M microelectrode recording –the section in the top left shows recording in a single level in a single channel; the pane in the bottom left shows the recording in the central channel over 11 mm and shows the typical firing pattern with irregular firing and broad baseline noted from -1.00 level; the top right shows the typical histogram frequency and the FFT signals of a typical STN neuron; the bottom right shows the same in a linear fashion.
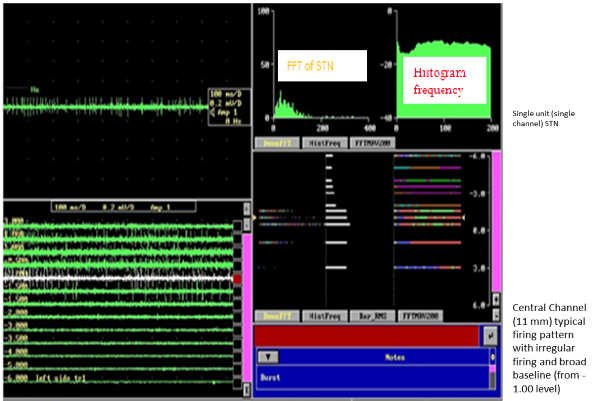
Phantom density with fast Fourier transform technique in frequency domain (FFT is an algorithm typically applied to compute the discrete Fourier transform DST with less number of additions and subtractions in order to estimate the spectrum) was computed using Framelink software and also in off-line Mat-Lab.
At the posterior level subthalamic-nuclei was encountered lower than expected, and at anterior it has come across higher than the expected and width of the nuclei was minute (Figure 3, Figure 4). The channel with maximum recording and the earliest recording were recorded on equal sides.
Clustering
Microelectrode recordings of these Parkinson patients were computed by applying principal-component tracking method and their feature values (mean ± SD) with DBS “on” and “off”. The PCs were solved for 12 PD candidates by using the Eigen vectors which were computed on a high speed Pentium computer.
Clustering Analysis is a method for grouping objects of similar kind into respective categories. Cluster analysis is an exploratory data analysis utility tool which aims at sorting different objects into groups in a way that the degree of association between two objects is maximal if they belong to the same group else minimal. Given the above, cluster analysis can be used to discover anatomical structures in data without providing an explanation—interpretation. In other words, cluster analysis simply discovers anatomical structures in the data without explaining why they exist. A further attempt at clustering these 12 patients by using the principal component (PC) scores of their “differences” in counts of signals were computed, with the following results: The total sum of squares is 89260 while the first two components account for 68836 = 50219 + 18617 i.e., accounts for about 75% of the variation in the data. This pair of coordinates give a good enough summary representation of the information conveyed by the distance matrix. The two (centered) principal component scores for the 12 patients are given in theTable 1.
Results and Discussions
Clinical diagnosis: 46 patients included in this study with mean age (58.1 + 9.1 years, disease duration 8.8 + 3.64 years), UPDRS score in off/on state (52.7 + 10.6/13. 4 + 5). Mean number of channels in which STN MER was detected (out of 5 multiple channels) on right side = 3.5+1.1, left = 3.6 + 1.04. 92 sides were computed. Figure 6 shows the percentage of people with the number of channels showing microelectrode recording.
Concordance rate with finer signal utmost width of recording was inferred. Final channel selected (central in 39/92 42.3%, anterior 31/92 -33.7%, medial 15/92 -16.3%, posterior 4/92 -4.3%, lateral in 3/92 -3.2%). Concordance with highest recording with chosen track was seen in 58.7%.62 Maximum length of (recording mean 5.3 ± 1.3mm, STN in the chosen track 48%), concordance with either highest recording or top length was noted in 64%. In 28 patients, final tract did not correspond either to the tract with top recording or most width of MER. 93.48% of patients showed STN recording in the final channel chosen. 13 patients had central tract, 8 had anterior, and 7 had medial as final tract. Mean length of MER recording in these channels was 2.3 ±1.8 mm. The final-tract chosen or preferred and majority was the central seen in 42.3% trailed with anterior in 33.7%. Concordance of last tract amid the channel containing the maximum-recording was 58.7%, with channel viewing paramount girth of recording (48%) and by means of each was64%. Dearth of any MER-signal-recording in the ending tract preferred was seen (in 6.52%). Out of the 6 PD candidates, one candidate had no signal-recording and probe was inserted in central-channel. Two candidates had medial, 2 anterior and 2 central-channels as their last-tract. And this was chosen by macrostimulation based on.
Prognostic—diagnosis: From the Table 1, it is experiential and experimental that the first two PCs are sufficient to précis and discriminating between the deep brain stimulator “ON” and “OFF” states and among the controls and different anomalies. The first Eigen-vector is best-mean-square-fit for the feature-vectors-of the twelve Parkinson subjects. Thus, PC1, i.e. the principal enormity of first Eigen-vector that explains the strength (amplitude) of the MER signal features in relative to the mean of the patients. We could recognize by visually inspecting the morphological second Eigen-vector, that second PC-emphasizes differences-between the right and left side variables. Indeed, the unilateral-onset and persistent asymmetry-of-symptoms support the diagnosis-of-PD in relation to other-similar-diseases.
Figure 5 depicting the principal component (PC) scores of the 12 Parkinson subjects, in which the points are well sprinkled away, devoid of lucid pattern but for the case of subjects {s6, s4, s7}. These three are close to one another as compared to the remaining nine PD subjects. Indeed, these three seem to form a lineal ordered set like radial curve and thus forming ellipsoidal curves or similar to clouds which are resembling like in the space with s4 coming between p6 and s7. It is also suggestive that {s3, s11, s12}, {s10, s5} may form two similar lineal ordered sets, though the distances are much larger than in the case of the first set. Patients {s8, s9} are isolated and are very farthest to each other and thus explaining 80% variance. However, these findings are to be cross—validated with clinical findings on the same patients. It is interesting that the D group patients do not form a cluster in this scenario. This perhaps needs further looking into the clinical picture of patterns, other than the C and D group—clusters.
We assessed the role of microelectrode stimulation in assortment of the last channel-amplifier. In contrast to the structural confinement on diagnostic imaging in which the last zone was observed in 42.3% (only) and microrecording be connected through last-channel in 64%, which is analogous to our earlier investigation in which by means of MER, a normal pass all the way through the STN of 5.6 mm was attained compared to 4.6 mm if central-tract was preferred as per MRI.
We state that the microelectrode-recording system is not an absolute utility—tool to noticeably differentiate the finest target as the row of electrode possibly might not matchup the alignment of the subthalamic-nuclei and the impedance of the micro-electrode could differ since they can be influenced by the tissue of the brain and might not demonstrate apparent micro-electrode-signal-recording. Nevertheless, micro-electrode-recording-system can be knowledgeable to corroborate the lucid point of the microelectrodes and strengthen the poise of the neurosurgeons to facilitate they are in the exact target.62
Conclusions
The prime objective of this study was to decode the predictive role of intraoperative neural signals in STN DBS response and provide scientific insights by revealing the most informative intraoperative MER features and extracting STN MER “patterns” associated with UPDRS improvement. We investigated the MER with STN high frequency DBS in PD, recorded the STN neural signals and applied PCA-based tracking method. Though results are partially meaningful clinically, these analyses could possibly be applied to longitudinal follow-ups, microanalysis and correlations with a normal control population in future to better comprehend the Parkinson`s disease with microelectrode-signal acquisition of the STN and also global pallidal (GP) neurons meaningfully. Absence of any recording from STN in the final tract selected was noted in 6/92 -6.52%. Out of 6 patients, 1 had no MER recording in any of the 5 channels and lead was placed in central channel. 2 had medial, 2 anterior and 2 had central channels as their final tract. This was selected based on macrostimulation. In our study, we achieved 75% variation in scatter plot and find that MER gives proof of correct-positioning of electrode, ensures accurate detection of STN precincts and determines its exact coordinates in a more objective way. MER enhances safety, accuracy and efficacy of DBS electrode implementation. Thus, MER confirms presence of abnormal STN neurons. Unperturbed MER definitely can confirm clear position of electrodes and bolsters the confidence of the neurosurgeons that they are in the target. Availability of MER results in a vast data regarding functioning on neurons situated deep in the brain may further help in unraveling mysteries of brain. This study correlated MER signal multiunit activity features with DBS improvement.